Innovative Diagnostics for Pediatric Leukemia at Notable Labs
Precision medicine has not only elevated the standard of care in routine clinical practice but also revolutionized cancer treatment. Notable Labs’ predictive platform, driven by cutting-edge machine learning, is at the forefront of this evolution, saving countless young lives diagnosed with rare cancers. This blog explores the transformative power of Notable Labs' predictive precision medicine platform, shedding light on its advanced technological foundations, life-saving impact in pediatric oncology, and the bright future it promises for personalized cancer treatment. As we embrace these innovations, we stand on the brink of a new era where every child diagnosed with cancer can hope for a brighter, healthier future.
Imagine a world where Medicine’s ultimate dream is accomplished- safe and guaranteed cancer therapies delivered swiftly, saving the lives of thousands. A world where physicians provide patients with novel cancer treatments tailored specifically to them, making rapid cures possible.
This vision is close to becoming reality, thanks to rapid advances in precision medicine. Leading the charge is Notable Labs, with its clinical stage predictive platform that’s revolutionizing routine cancer care. Leveraging advanced computational capabilities and machine learning algorithms, Notable Lab’s platform predicts the most effective treatments for pediatric cancer. This integrated approach has delivered assured therapeutics rapidly to children with cancer. With an impressive 95% prediction accuracy that was demonstrated in a Stanford study, Notable’s platform is poised to transform cancer therapy and save countless lives, redefining the trajectory of pediatric cancer care in real time.
Pediatric Leukemia is a Rare Disease
Childhood leukemia is the most common cancer type in children. According to the National Cancer Institute (NCI), 1 out of 3 childhood cancers is a leukemia subtype, and 112,658 cases of pediatric leukemia were registered in the US in 2020[1]. The overall survival rates for pediatric leukemia have improved, but the prognosis remains grim for rare forms like pediatric acute myeloid leukemia (pAML) and juvenile myelomonocytic leukemia (JMML).
Since pediatric AML and JMML leukemia subtypes are rare, they present unique challenges to clinical trials. Conventional clinical trials test a drug by grouping patients under a single disease ‘umbrella’. This approach has produced highly safe and effective drugs, but complicates cancer therapy, as:
- A single drug/drug combination is tested in Phase2 on a select clinical population (Phase1 ensures drug safety before testing with patients in Phase2). This means that some of the drug’s indications could be masked, simply because they didn’t apply to the trial patients.
- A very complex variable that significantly changes treatment outcomes gets overlooked- the individual’s cancer profile.
Technical Background
Precision Medicine: Overcoming Challenges in Leukemia Treatment
A patient’s unique biology affects their drug sensitivity and response. Conventional trials that validate drug interventions don’t account for individual factors, such as genetics, which can significantly impact and alter the trajectory of a drug’s therapeutic outcomes.
In contrast, precision medicine leverages an individual’s genetics to tailor an intervention that addresses individualistic variations in drug sensitivity and response. Databanks such as The Cancer Genome Atlas (TCGA) and International Cancer Genome Consortium (ICGC) collect robust data of various genetic mutation profiles, associated with specific cancer subtypes. For example, some cancer mutations (TP53, PIKCA [2]) are common across many cancers, whereas acute myeloid leukemia (AML) is associated with actionable genetic mutations in FMS-like tyrosine kinase 3[3] (FLT3). (Actionable mutations refer to gene variations with known and safe drug interventions).
Combining genomic databank knowledge with an individual’s clinical data (biopsies, blood tests, biomarkers, etc.) transforms clinical medicine into precision medicine. Researchers deploy the latest in big data analytics, to leverage the combined clinicogenomic data, so that doctors can tailor a treatment to the patient in real-time by pinpointing exactly which signaling pathways have been disrupted.
For example, midostaurin is a drug identified by precision medicine that targets a specific AML mutant of FLT3, called FLT3-TKD (Tyrosine Kinase Domain) [3]. Quizartinib is a precision medicine drug that inhibits a different FLT3 mutant: FLT3-ITD (internal tandem duplication), and does not target FLT3-TKD. This means that a cancer patient with FLT3-TKD mutation would not respond to quizartinib. This reinforces how huge an impact the individual’s unique cancer biology has on drug outcomes.
Precision Medicine in Pediatric Leukemia: Current Challenges
Two formidable challenges limit the implementation of precision medicine in routine clinical practice for pediatric leukemia: The quality and quantity of genomic data radically impacts its predictive power.
Targeting actionable gene mutations has significantly improved treatment outcomes; yet, the genetic data itself can become a constraint. For example, a drug identified through precision medicine, gilteritinib, targets an FLT3 mutation in AML [4]. Yet, only 34% of FLT3+ patients respond to the drug.
Several factors could contribute to low drug efficacy:
- Selection of a clinical trial population: Lack of genetic diversity in the selected population can cause large gaps in causal data. This missing data cannot be extrapolated, since ethnic and genetic diversity in disease pathogenesis cannot be predicted without adequate representation of such diversity.
- Rare diseases: when cancer incidence is low, such as in pAML or JMML, fewer individuals with unique cancer biology are represented in a clinical population.
- The predictive power of genomic data alone is severely limited, since cancer is multifactorial
Harnessing the complete genetic profile of a cancer may increase treatment predictive power, but still limits drug efficacy, since cancer biology is multifactorial.
Apart from genetics, an individual’s lifestyle, environment, metabolic health, cellular health, nutritional health, proteome, transcriptome, microbiome, history, immunity, and other factors- significantly impact patient response to treatment.
The multifactorial nature of pediatric leukemia, and the low incidence of rare cancers, restrict the application of genome-targeted precision medicine. This is evidenced by studies that evaluate genome-targeted therapy for cancers. Precision treatment efficacy was found to be 7.04% in 2020 for cancers- a significant improvement from 3% in 2016,[5] but still very low.
Precision medicine trials that collect multifactorial data are thus extremely crucial for redesigning cancer care. Platform trials, such as the pedAL trial by the Leukemia and Lymphoma Society [6] (LLS), continue to improve their data collection approaches.
The ongoing pedAL master trial conducts both genomic testing and cytogenetic screening for enrolled patients, to account for individual differences in genetics and cell biology. The pedAL trial emphasizes the importance of molecular biomarkers in guiding therapy choices, and closely aligns with Notable's own diagnostic methodology.
Notable Labs Approach
Notable Labs’ Approach: Predictive Medicine in Transforming Cancer Treatment
Notable Labs’ predictive precision medicine platform (PPMP) is a clinical stage therapeutic platform that’s revolutionizing pediatric leukemia treatment. Notable’s platform predicts an individual’s unique biological response or drug sensitivity, by integrating machine learning algorithms and comprehensive biological data analysis. A pivotal study, by researchers at Stanford University, demonstrated that Notable’s predictive PMP achieved 95% accuracy in predicting effective treatments for pediatric leukemia.
Patient-specific responses are calibrated to multiple drugs, using high-throughput screening and advanced computational models. The typical turnaround time for Notable’s PPMP is one week. The platform prioritizes a safe, targeted approach to cancer care, minimizing the probability of adverse drug effects. Notable’s platform scores and ranks the likelihood of various interactions within a patient’s cellular environment that could increase adverse effects, thus guiding treatment options real-time.
Notable’s Platform Predicts 95% Treatment Outcomes
Notable’s PPMP, referred to as functional drug-sensitivity screening (DSS) platform in journal publications, was validated by four different clinical trials conducted by Stanford University, Washington University, and the Texas’ Children Cancer Hospital.
In the Stanford validation trial [7], 21 patients whose leukemia subtype had proven recalcitrant to prior chemotherapy treatment were administered Notable’s platform-screened drugs. The PPMP accurately predicted 95% of the patient’s response to treatment.
This exponential capacity of Notable’s PPMP is thanks to its unique approach in deploying functional precision medicine, or predictive medicine, to identify drug candidates. The PPMP integrates patient information to rank treatments based on the patient’s predicted response to a drug. The predicted response is represented as a function of the cancer’s positive predictive value (PPV) (PPV is used in clinical trials, and is the ratio of true cancer positives to false positives). By calibrating Notable’s platform predictive score to an existing cancer ratio, readouts are easily interpreted to inform clinical decisions in real time.
Clinical Outcomes
Notable’s Platform Successfully Guides Treatment of Rare Pediatric Cancers
Notable Labs has forged successful collaborations with leading institutions to ensure that its platform’s transformative predictive power can reach patients in real-time. Notable’s collaborators include oncologists from Texas Children’s Cancer Hospital, and Dr. Elliott Stieglitz from the University of California San Francisco (UCSF).
We provide below, a few examples of Notable’s success in guiding real-time clinical decisions.
Notable’s PPMP successfully tailored a repurposed drug to treat cancer.
Notable’s PPMP has proven instrumental in prescribing life-saving therapies for many children in real-time. One noteworthy case involved a young patient at the Texas Children’s Hospital, diagnosed with pediatric acute myeloid leukemia (pAML)- a rare, aggressive leukemia that averages 500 cases in the US every year[8]. The patient was administered a regimen of traditional chemotherapy options, which included ADE (A-Cytarabine, Daunorubicin, Etoposide). Chemotherapy, however had limited success, and the patient's condition began deteriorating rapidly.
Utilizing Notable’s platform, the medical team identified a repurposed adult drug (Atovaquone) with a high predictive value for the specific patient's leukemia subtype. The treatment resulted in a remarkable improvement, leading to complete remission. The treatment’s resounding success redefines cancer care, reinforcing functional precision medicine as pivotal to streamlining targeted therapies for rare cancers.
Notable Labs identifies novel therapeutic combinations that enhance chemotherapy
In another successful case study, Dr. Elliott Stieglitz at UCSF collaborated with Notable Labs to explore therapeutic directions for JMML, a second rare and challenging pediatric leukemia subtype [9]. Treatment for JMML is hematopoietic stem cell transplant (HSCT), prior to which moderately intensive chemotherapy is given. However, pre-HSCT chemo does not completely eliminate cancer cells, and patients typically proceed for transplantation with active malignant cells.
Notable's PPMP screened 130 drug combinations against bone and blood marrow samples from JMML patients, and discovered a drug that intensified pre-HSCT chemotherapy to be more effective. Interestingly, the same drug had effects on cancer cells that could be exploited to enhance novel treatment routes for pre-HSCT treatment.
Notable’s PPMP Pinpoints Novel Specific Drug Combination for pAML Treatment
A clinical study conducted by the Texas’ Children Hospital deployed Notable’s platform, to predict the most efficient treatment for pediatric AML from 77 treatment conditions [10]. Notable’s platform identified a completely novel drug combination, Bortezomib and Panobinostat (B/P), as an effective treatment for multiple pAML subtypes. In addition, Notable’s PPMP accurately identified one patient’s insensitivity to conventional drug combination therapy, and accurately predicted their sensitivity to B/P treatment [10].
These instances emphasize the necessity and relevance of leveraging predictive medicine in real-time patient treatment. Notable’s predictive PM platform plays a crucial role in identifying novel treatment pathways, showcasing the power of precision diagnostics in guiding research and clinical decisions. The PPMP’s technological capacity to accurately predict and delineate patient responses to multiple leukemia treatment options could transform many young lives.
Drug Development
Notable’s PPMP Advances Drug Trials in Pediatric Leukemia
An exciting development of Notable’s predictive platform is in its potential application to Volasertib, a drug targeting PLK1. PLK1 or Polo-like-kinase is implicated in leukemia cell proliferation. Volasertib is a drug that targets PLK1 activity [11], with demonstrated capacity to improve AML outcomes in conventional Phase2 clinical trials.
Clinical trials have found that a combination of Volasertib with low-dose cytarabine (LDAC), an established treatment for AML, increased the overall treatment response from 13.3% to 31% [12]. However, Phase3 trials were less successful, faced with challenges due to inconsistent antibiotic management of post-chemotherapy infections. Boehringer-Ingelheim, the pharmaceutical giant conducting the clinical trial, is currently conducting an enhanced Phase2 trial with appropriate antibiotics measures.
In an exciting development, Notable Lab’s predictive PM platform will enhance Phase2 trials, by accurately identifying patients responsive to Volasertib [13]. The goal is to identify patients sensitive to Volasertib in Phase2, so that Phase3 will be an accurate representation of a drug-responsive population.
By successfully predicting patient sensitivity to Volasertib in Phase2 clinical trials, Notable’s PPMP will be opening exciting new avenues that accelerate the provision of guaranteed cancer therapies to young patients.
Key Highlights
Pediatric Leukemia Statistics
112,658 patients with pediatric leukemia were registered in 2020 (According to the National Cancer Institute). 49.2% of childhood cancers are leukemias, with a higher incidence in male children (54.4%).
Survival Rates
The 5-year relative survival rate is 87% (According to the National Cancer Institute). Overall survival rates for leukemia have improved, but challenges remain for rarer forms. The survival rate for pAML is 70%, and JMML is approximately 79%.
Shift to Precision Medicine
Traditional clinical trials are limited in their scope due to the multifactorial nature of cancer. Precision medicine offers a streamlined approach, tailoring drug interventions to an individual’s unique biology. Notable Lab’s platform enhances precision medicine approaches to expand the pediatric leukemia treatment landscape.
Success Stories
The Stanford study corroborated Notable’s 95% prediction accuracy for effective treatments. Notable’s PPMP platform successfully prescribed life-saving therapies in young patients, identifying specific drugs and drug combinations in the treatment of pAML and JMML.
Collaborations
Texas Children’s Hospital and University of California San Francisco (UCSF)
Potential in Accelerating Drug Development: Volasertib Phase2 enhanced trials will be further augmented by PPMP’s demonstrated capability in predicting patient responsivity to the drug. With Notable’s platform, volasertib enhanced Phase2 trials will be able to distinguish patient factors that impact drug sensitivity, enhancing worldwide Phase3 trials.
Conclusion
Notable’s predictive platform has visibly brightened the future of cancer care, becoming the disruptive technology in routine precision medicine. Future treatments for pediatric leukemia are even now being reoriented to Notable’s platform; for example, the data on JMML drug responsivity that Notable Labs curated in collaboration with UCSF, will be leveraged by clinical physicians to prioritize therapeutic options and care practices for young patients.
Notable Lab’s groundbreaking predictive platform offers hope for assured and personalized treatments, paving the way for a new era in cancer care. The predictive precision medicine platform aligns with the current trend in clinical trials, which is shifting towards patient-centric narratives. Notable’s PPMP is designed to embrace and enhance the shifting trend, and multiplies the benefits of early functional drug sensitivity testing. To illustrate, platform trials like pedAL are creating a “patient atlas” that would exponentially magnify the PPM platform’s predictive power.
Notable’s PPMP pipeline neatly integrates into routine clinical practice with a turnaround of one week, easily guiding real-time clinical decisions and targeted treatment. By harnessing the power of ML-algorithms and comprehensive biological data, Notable Labs is setting new standards in precision medicine, ensuring that each child receives the best possible care.
With cancer therapeutics accelerated through clinical pipelines, Notable Labs heralds a reimagined future for delivering precision medicine in pediatric cancer care. As we look ahead, the integration of Notable’s advanced predictive technologies into routine clinical practice promises to improve survival rates and quality of life for young patients worldwide, bringing us closer to a world where cancer is not just treatable but curable.
References
- National Cancer Institute. NCCR*Explorer: An interactive website for NCCR cancer statistics. Internet. (2023).
- Sinkala M. Mutational landscape of cancer-driver genes across human cancers. Sci Rep. 7;13(1):12742 (2023).
- Heuser, M., Mina, A., Stein, E. M. & Altman, J. K. How Precision Medicine Is Changing Acute Myeloid Leukemia Therapy. Am Soc Clin Oncol Educ Book 39, 411–420 (2019).
- Perl, A. E. et al. Gilteritinib or Chemotherapy for Relapsed or Refractory FLT3-Mutated AML. N Engl J Med 381, 1728–1740 (2019).
- Haslam, A., Kim, M. S. & Prasad, V. Updated estimates of eligibility for and response to genome-targeted oncology drugs among US cancer patients, 2006-2020. Ann Oncol 32, 926–932 (2021).
- The Leukemia and Lymphoma Society. The LLS PedAL Master Trial: A Clinical Trial for Acute Myeloid Leukemia Pediatric Patients. (2025).
- Spinner, M. A. et al. Ex vivo drug screening defines novel drug sensitivity patterns for informing personalized therapy in myeloid neoplasms. Blood Adv 4, 2768–2778 (2020).
- Notable Labs collab. with Texas Children’s Cancer Center. Ex vivo drug sensitivity assay correlates with clinical response in pediatric AML. Annual Meeting American Society of Clinical Oncology (ASCO) (2021).
- E. Stieglitz et al., ‘Tretinoin Enhances the Effects of Chemotherapy in Juvenile Myelomonocytic Leukemia Using an Ex Vivo Drug Sensitivity Assay’, JCO Precis Oncol 7, (2023).
- Strachan, D. C. et al. Ex Vivo Drug Sensitivity Correlates with Clinical Response and Supports Personalized Therapy in Pediatric AML. Cancers (Basel) 14, (2022).
- Van den Bossche, J et al. Spotlight on Volasertib: Preclinical and Clinical Evaluation of a Promising Plk1 Inhibitor. Medicinal research reviews 36,4 (2016).
- Döhner, H. et al. Randomized, phase 2 trial of low-dose cytarabine with or without volasertib in AML patients not suitable for induction therapy. Blood 124, 1426–1433 (2014).
- Notable Labs. Guided by a predictive ex vivo test: bringing the PLK1 inhibitor volasertib back into the clinic for relapsed/refractory acute myeloid leukemia patients. Presented at AACR 2024, San Diego, CA AACR 2024, abstract published in Cancer Res 84,(6_Supplement): 5178 (2024).
About the Author
Divya Narasimhan, MSc | Science Communicator in healthcare and biology Masters With Research in protein & biomedical science Divya has 3+ years’ writing experience in science communication, and brings her research expertise to creating science blogs for a wide readership. She graduated from the National University of Singapore, and enjoys working with physicians and scientists in the biomedical field to showcase innovative products & technologies that redefine healthcare and science.
Disclaimer
The content of these blogs reflects the research and opinions of the individual authors and does not necessarily represent the views or positions of Notable Labs or its affiliates. The information provided is for educational and informational purposes only and should not be construed as medical, legal, or financial advice.
Notable Labs makes no representations as to the accuracy, completeness, or validity of any information in these blogs and will not be liable for any errors, omissions, or any losses, injuries, or damages arising from their use.
These blogs may reference third-party research, studies, or resources. Notable Labs does not endorse or assume responsibility for the content or practices of these third parties. Any reliance on the information provided is at the reader's own risk.
For biotechnology and pharmaceutical content, note that ongoing research and clinical trials may change the context and results discussed. Always refer to the latest research and guidelines from reputable sources.
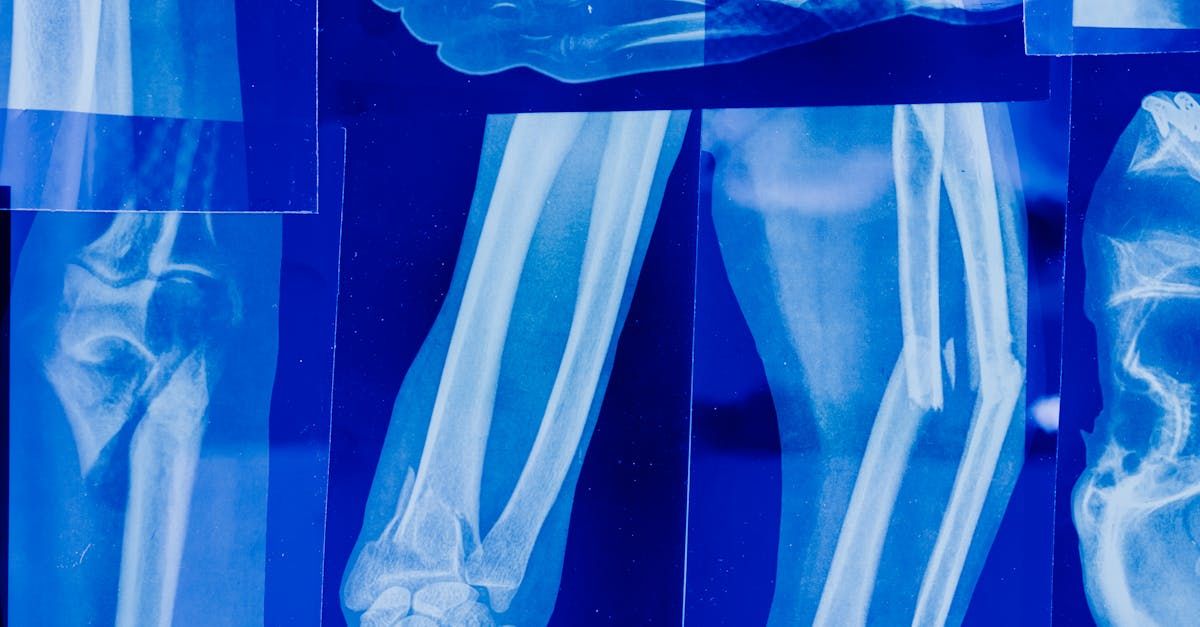
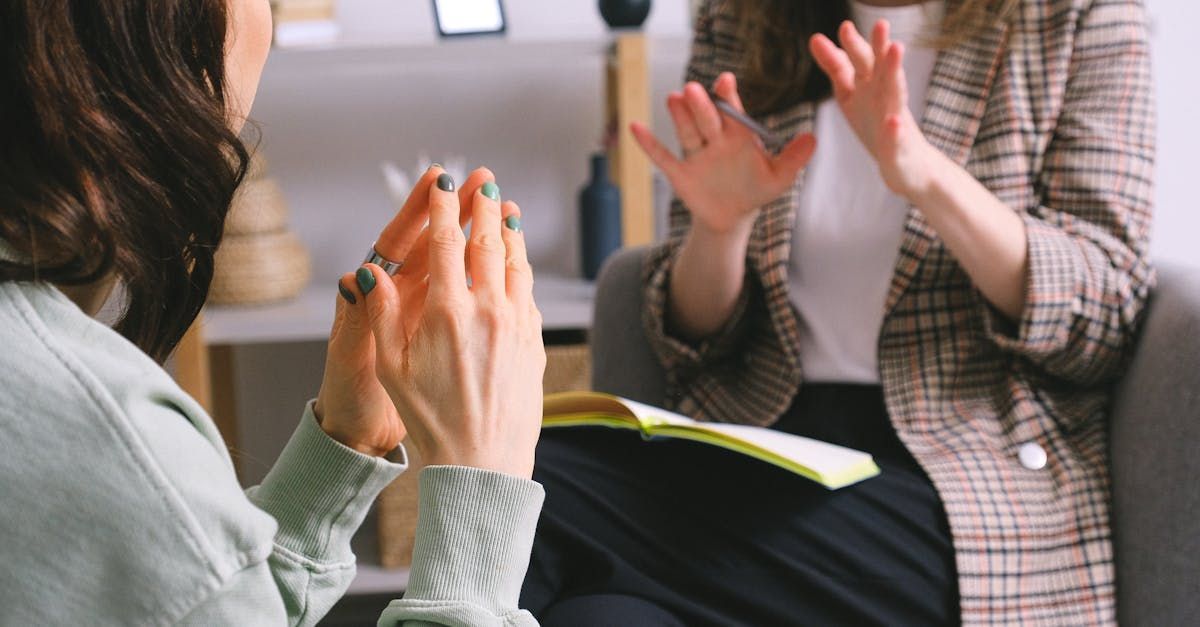
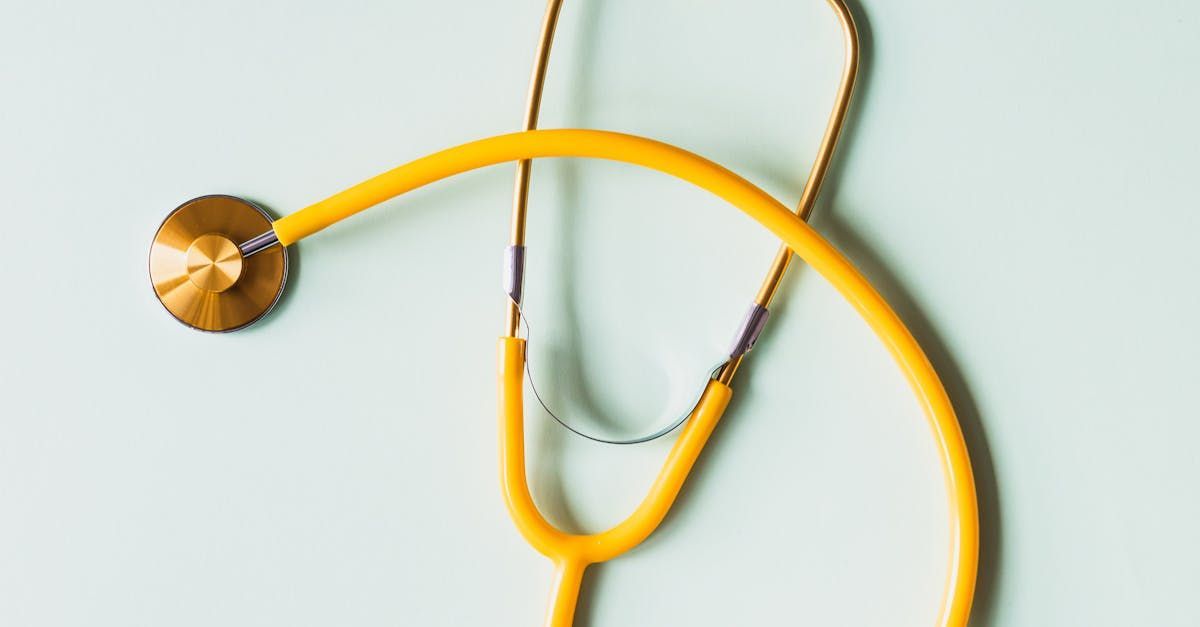
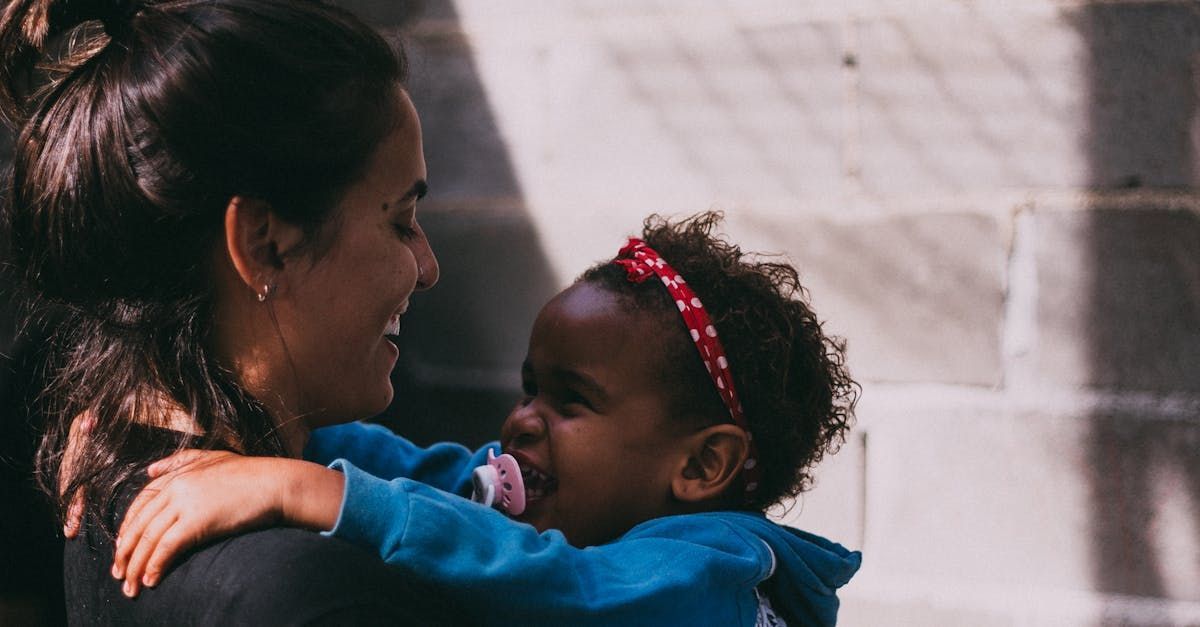
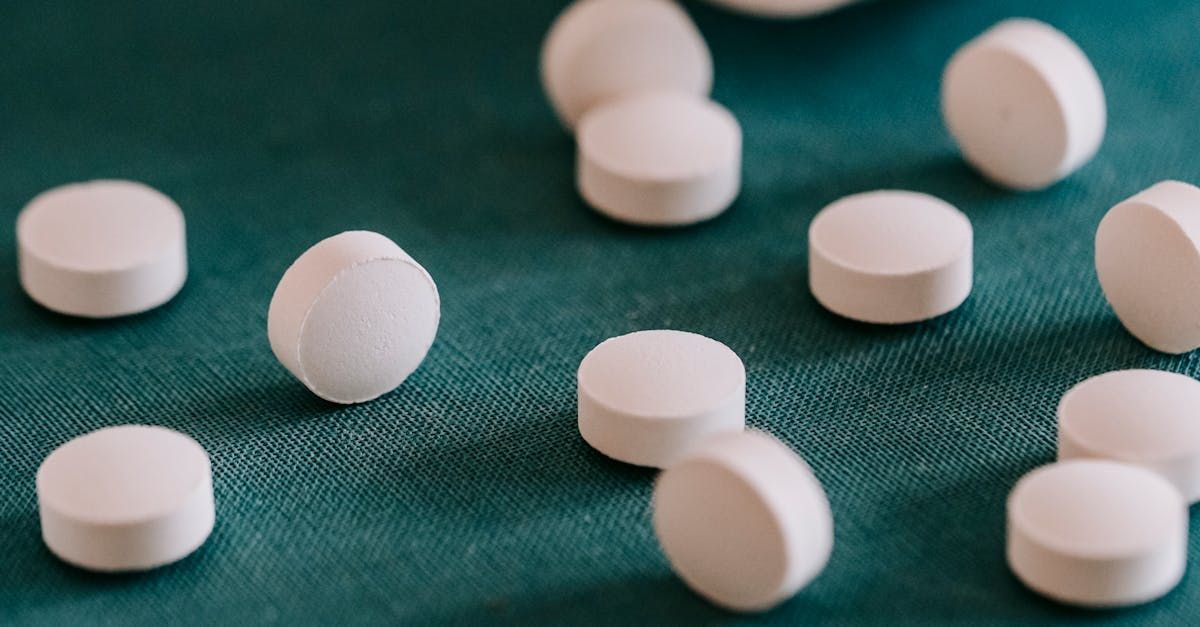
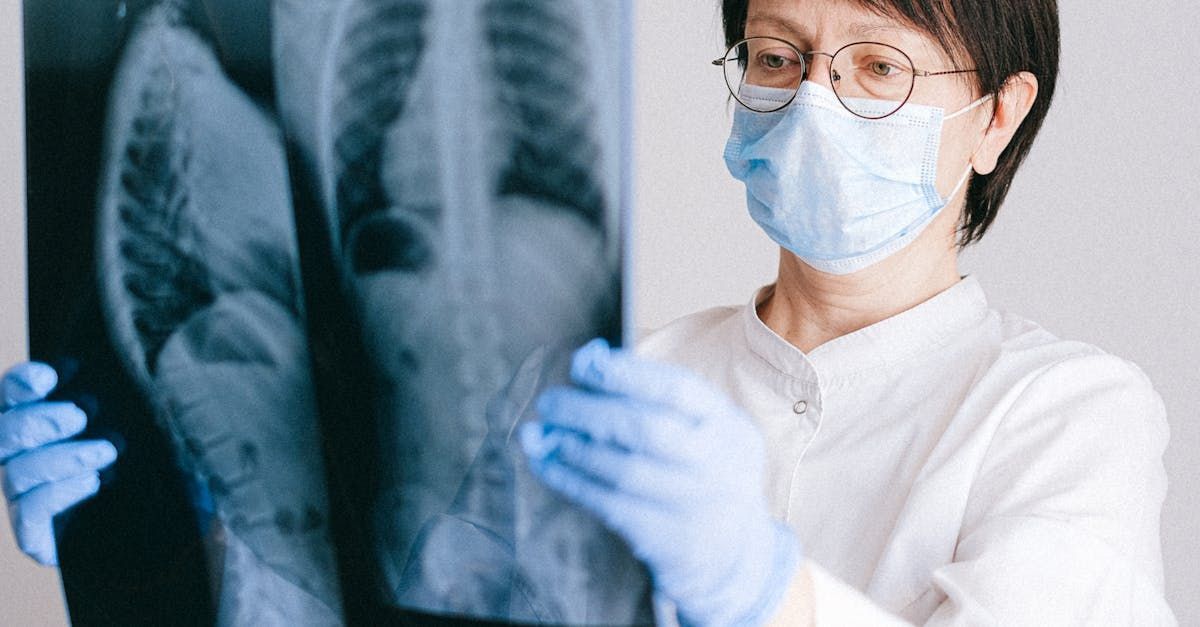
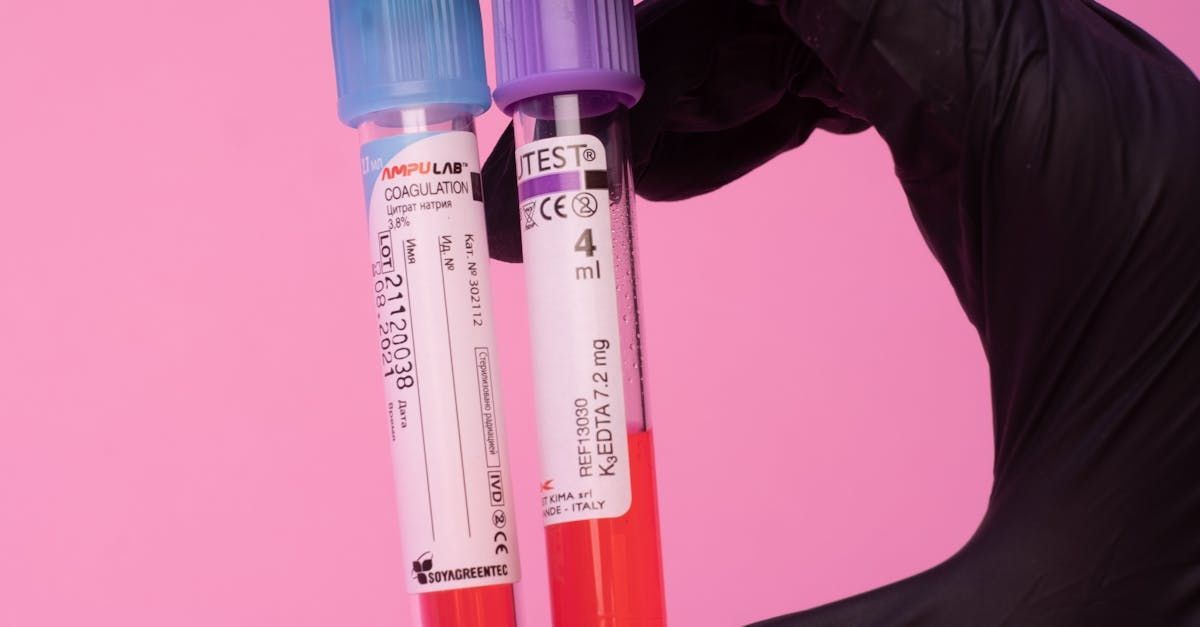
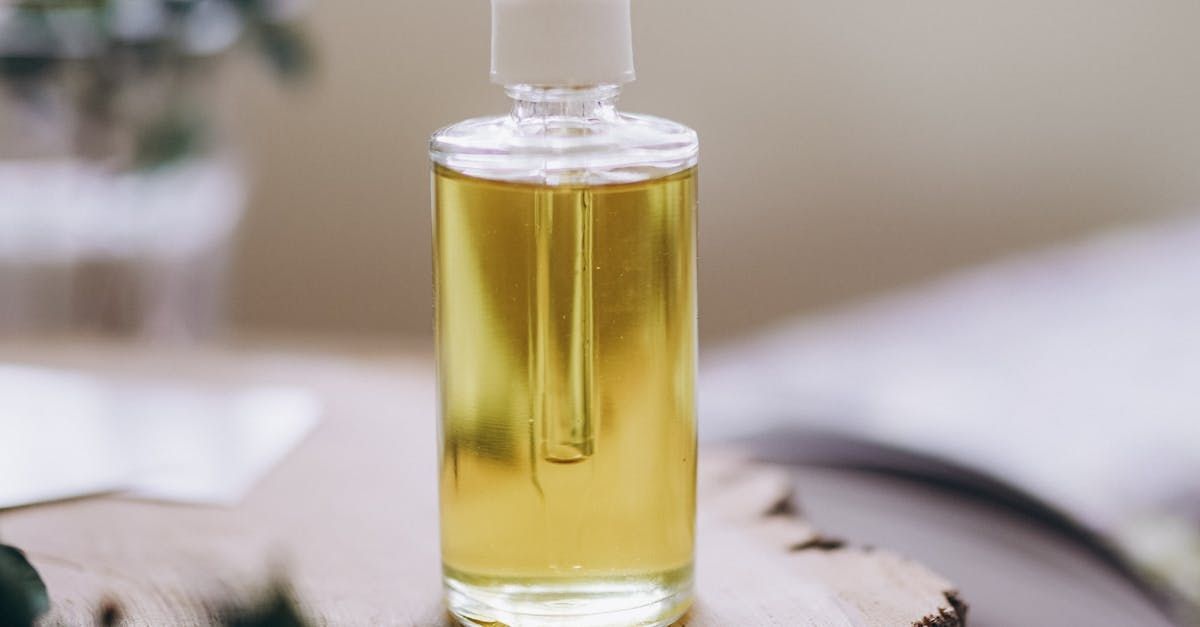
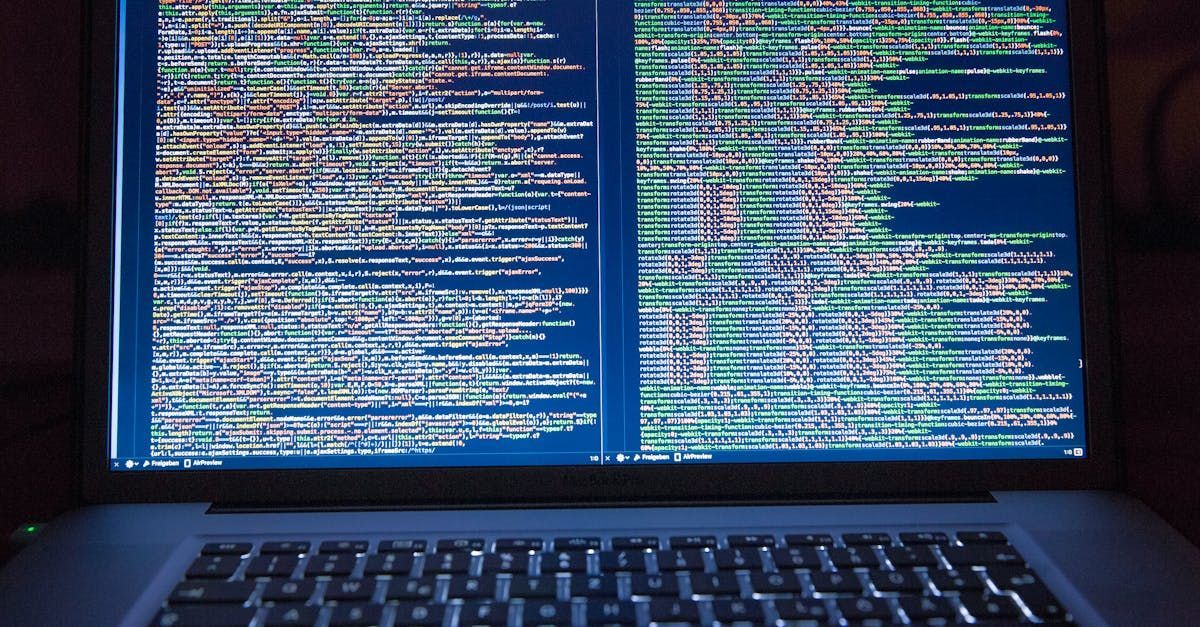
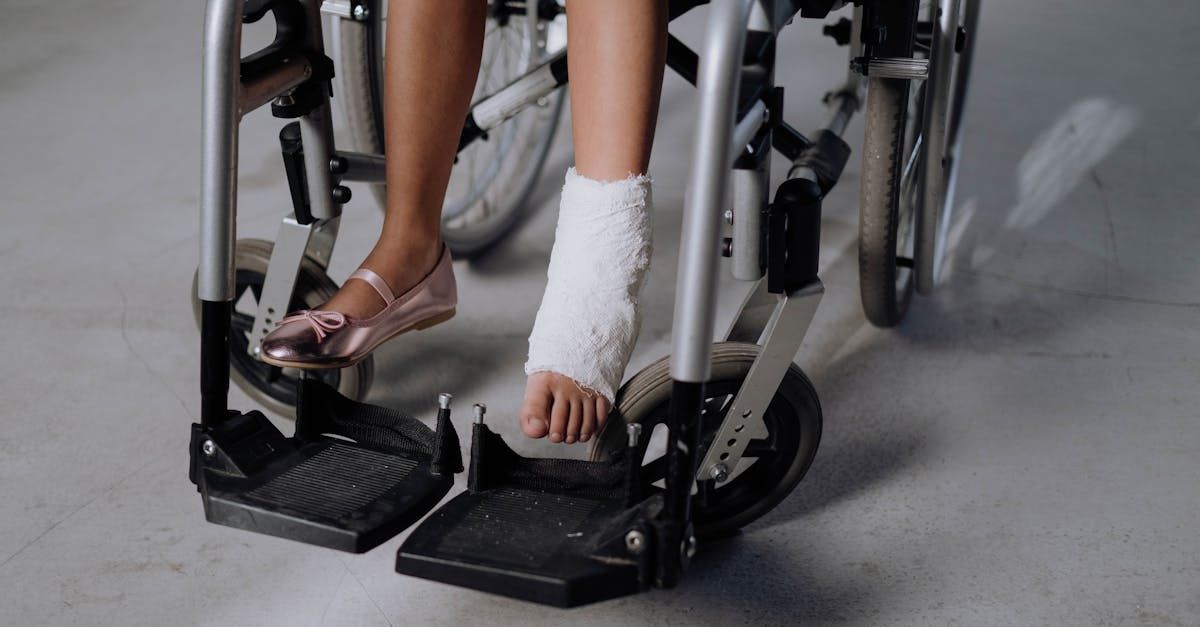